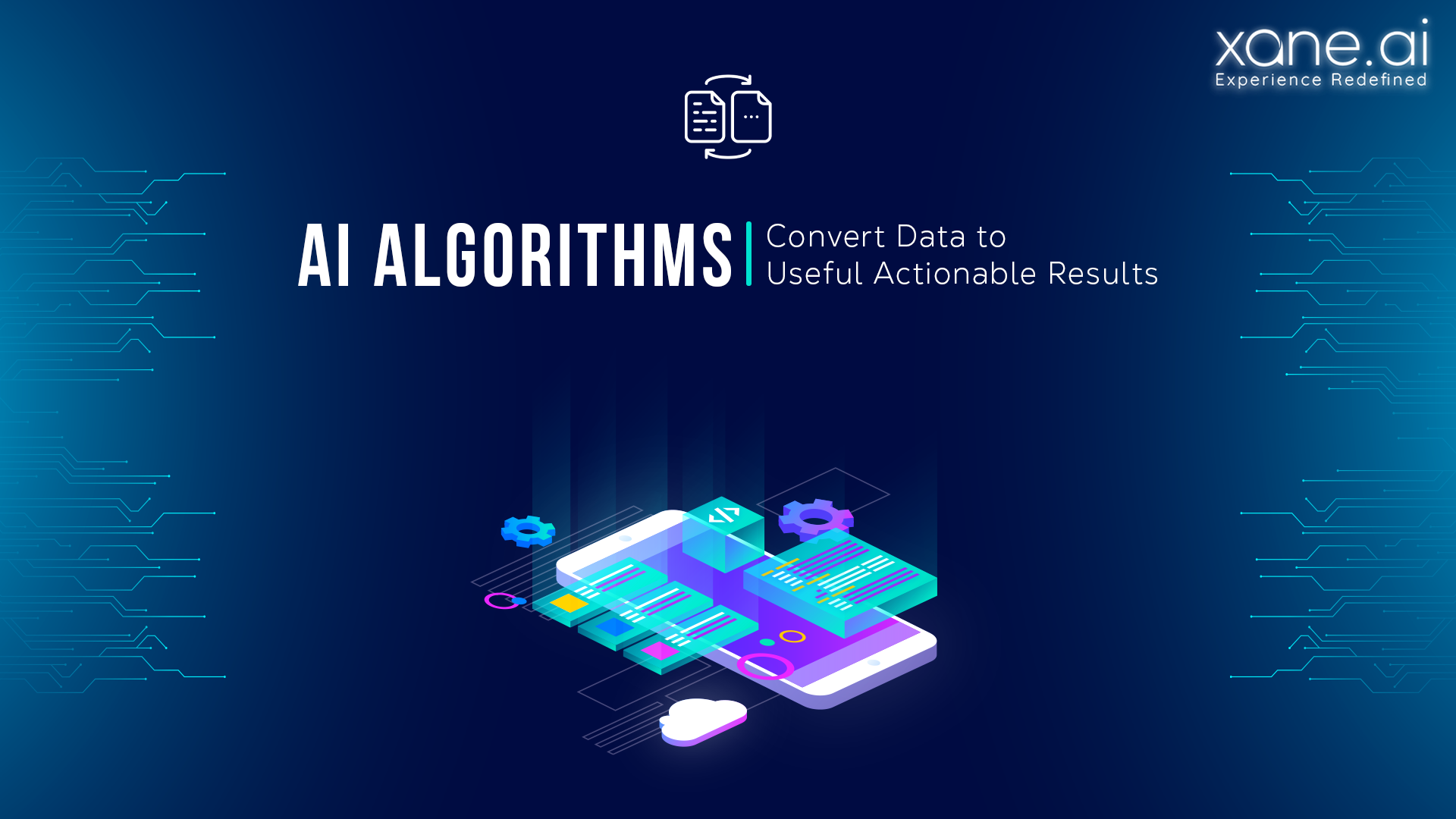
30 May Artificial Intelligence Algorithms Convert Data to Useful Actionable Results
Blog by Oluwasegun Oke
The day’s computers were merely pre-programmable systems when trained and certified algorithm experts were always on standby, to correct anomalies, implement insights and help advance computer decision-making processes, which had long taken a new perspective, since the inception of machine learning – a vital field in artificial intelligence.
It has over the years, wisely conceptualised how learning algorithms expansive tools are being applied in troubleshooting potential defections, and other parameters that may affect the optimum performance of computer systems, such that automatic processes are in place to override inconsistencies, and put distressed operations back to normalcy.
This field, known as machine learning is prominent and provident, by measuring and determining internal structures of algorithms, while forecasting or making decisions, to discern what comes next, during various computer tasks, for enhanced performance and guaranteed actionable results.
It gives the machine an edge to learn both from experience, and while in operation, as it sifts through different orientations of patterns, to learn and understand certain behaviours, in order to function independently, in accordance to present needs, as against intermittently being manually corrected by a standby operator. It encompasses all well-planned algorithms, of different constituents, through models, which enable computers to learn unprompted – just like humans.
Training of Models
Algorithm models are behind every decision-making process. Computers perform on daily basis, with the help of system dynamics. These vital models have learned from many past and present training, including automated big data tools, to become a standard for understanding datasets, detecting patterns, and processing results for a wide range of operations.
The following are the steps in training algorithm models:
- Introduce new sample data into a computer.
- Test if the data have been entirely scanned, extracted, and made a signature (model).
- Input a replica of the instruction and see if it is able to detect what it represents.
- If detected and validated, it has learned it successfully. If not try again.
- Now introduced another sample of profiling data, and evaluate how fast and accurate, it was able to compare both internal features and patterns, so as to extract and identify each of their resemblances matching its components.
The above processing is known as training, it allows learning algorithm models to become more effective and provident, by regularly updating their designs to enhance their self-programming capabilities. By doing so, no outside efforts are required to advance any computer operations, under whatever circumstances. It empowers computer systems to automate processes, detect inconsistencies, and provide solutions without any algorithm expert’s interventions.
Given the ambiguities surrounding the description and meaning of data, we shall discuss further its attributes along with what both information and insights, stand for, as defined through data science.
Data
Data refer to the configuration, or a bunch of items, or order of formations of entities, which are in their raw states, with no further nor specific meanings. In essence, they are unprocessed and may either be quantitative (measurements), or qualitative (observations).
Information
Information connotes a group of data, which have been processed, collected, and arranged into structural patterns which can easily be understood by humans.
Insights
Insights take shape as both data and information are looked into, and analysed, in order to comprehend the context of a situation and events, such that it provides sufficient metrics and facts, which can be used to draw conclusions. More so, insights can be actionable and un-actionable, the former offers information towards a solution, causing a purposeful and meaningful directional action to be performed, especially in taking business-oriented decisions. While the latter only provides you with isolated information, that does not help in taking action, let alone reaching any conclusions.
The major attributes of insights are as follows:
Specificity
The more unspecific a data is, the more isolated, meaningless, and un-useful it becomes. That said, data may later become informative enough to pick up providence and offer valuable actionable solutions, upon further analysis and development. It, therefore, lays higher emphasis on automation and prompt recovery from every algorithmic indecision, after an error, misjudgment, and other unforeseen issues.
Relevance
A concept that only resonates around the right audience. In essence, delivering the right data to the wrong audience renders insights inactive, and obliterate such campaigns. The need for relevance, therefore, encompasses using the right tools and environment as well, in a timely manner, such that your desired results become feasible.
Alignment
Not positioning your insights towards your company’s visions, goals, and aspirations can prove unwise. This may eventually hit back, stalling business visions, by indefinitely postponing such unaligned priorities. It is, therefore, wise to align insights with regard to current business needs.
Context
Context is essential in making insights into what they really are. Without context, data is just a set of numbers or empty buzzwords. It makes insights more unique for its interpretations, to maintain originality, and keep out vagueness.
Novelty
Eccentricity sometimes holds empowering visions and colossal amounts of creativity. As human nature often demands probing unusual or newly discovered trends, in order to face and overcome business challenges. Therefore novelty is a tool for insights to evolve and adapt to different actionable circumstances, and more importantly to new settings of life.
Clarity
It fully portrays and dictates the internal, external, and otherwise structuring, of insight, including its meaning, for timely actionable decisions to be formulated, and implemented over time. Its absence in a business venture, amounts to wasted hours, with little comprehension of colossal data, leading to confusion and frustration.
Having explained the foundations of data, information, and different attributes of insights, however, at this point onward, we shall refer to actionable insights as “actionable data”, so as to be more aligned with general view and consensus.
Therefore, actionable data symbolise everything that comes to mind, whenever thoroughly outlined and clearly defined statistical digital arrangements, of every state, government, bank, insurance, security enforcement agency, and other institutions’ biometric identities, are brought up. And are critical in all ramifications of human existence. All these entities can be used to take valuable actions and drive a meaningful business decision, with no further ado.
Actionable data is in colossal amounts in many privates and public organizations. They are practically lying away somewhere, each time they are collected. This is because only about 29 percent are successful at remaining analytic data-driven, while up to 78 percent do not interpret nor process such useful information, to draw business insights, and make sales.
In the same way, a good campaign using the analytic models by a business venture, to understand customers’ past and present behaviors, through identifying similar patterns, and common interests, can help to deduce certain metrics and reach the perfect initiative to explore and reap myriads of business benefits, while enhancing customers experience.
At this crossroad, there are two types of actionable data:
- Direct actionable data
- Indirect actionable data.
Direct Actionable Data
It is specific and towards a particular event. Also, it is usually about one or more individuals, who have a stake, interest, or whose participation is requested or critical to completing a particular cause, and related campaigns.
For instance, a new app may require users to test its awesome features and overall functionality. Likewise, an insurance company may send a reminder text message to its staff to ensure they observe all directives inclusive in a previously circulated memo. Also, by email, a university may prompt pre-selected students to participate in an online survey.
Indirect Actionable Data
In contrast, indirect actionable data is often deduced from internally reached long-term prospects to conceive a plan, through predetermined data analysis, towards envisioned strategies, which are then pursued, to achieve a better decision-making process, and high return on investment.
The design and scope of actionable data follow certain traits and principles which are summed up below.
Accuracy
It represents the first step towards attaining a data-driven business decision-making process. And encapsulates a few categories of data elements, as follows:
- Authenticity
- Validity
- Clarity
- Equality
Accessibility
For actionable data to be designed and put to use, its accessibility is uncompromisable, and all properties guiding it are the following:
- User Capability
- Data Modalities
- Design Modalities
Ubiquity
They are downloadable and accessible through SaaS brands’ programming application interfaces. It is the attributes of machine-readable data, that aids in the transferability and availability of data within and between computer algorithm systems. And its data elements are as listed below:
- Perseverance.
- Integration.
- Virtual Availability
Quality
It lays emphasis on data’s validity throughout every campaign. Its thoroughness, completeness, security, include being able to be arranged into smaller indivisible units. That said, it all goes a long way in determining how reusable and functional such databases is to improve business services.
Organization
Data have to be well sorted out and meaningfully arranged, in order to become useful and actionable. It remains a providence and ever foremost context, revolving around the designing and implementation of algorithm models. And next are its five basic data elements:
- Connotation
- Updated Taxonomies
- Dynamic Parsing
- Context
- Logic