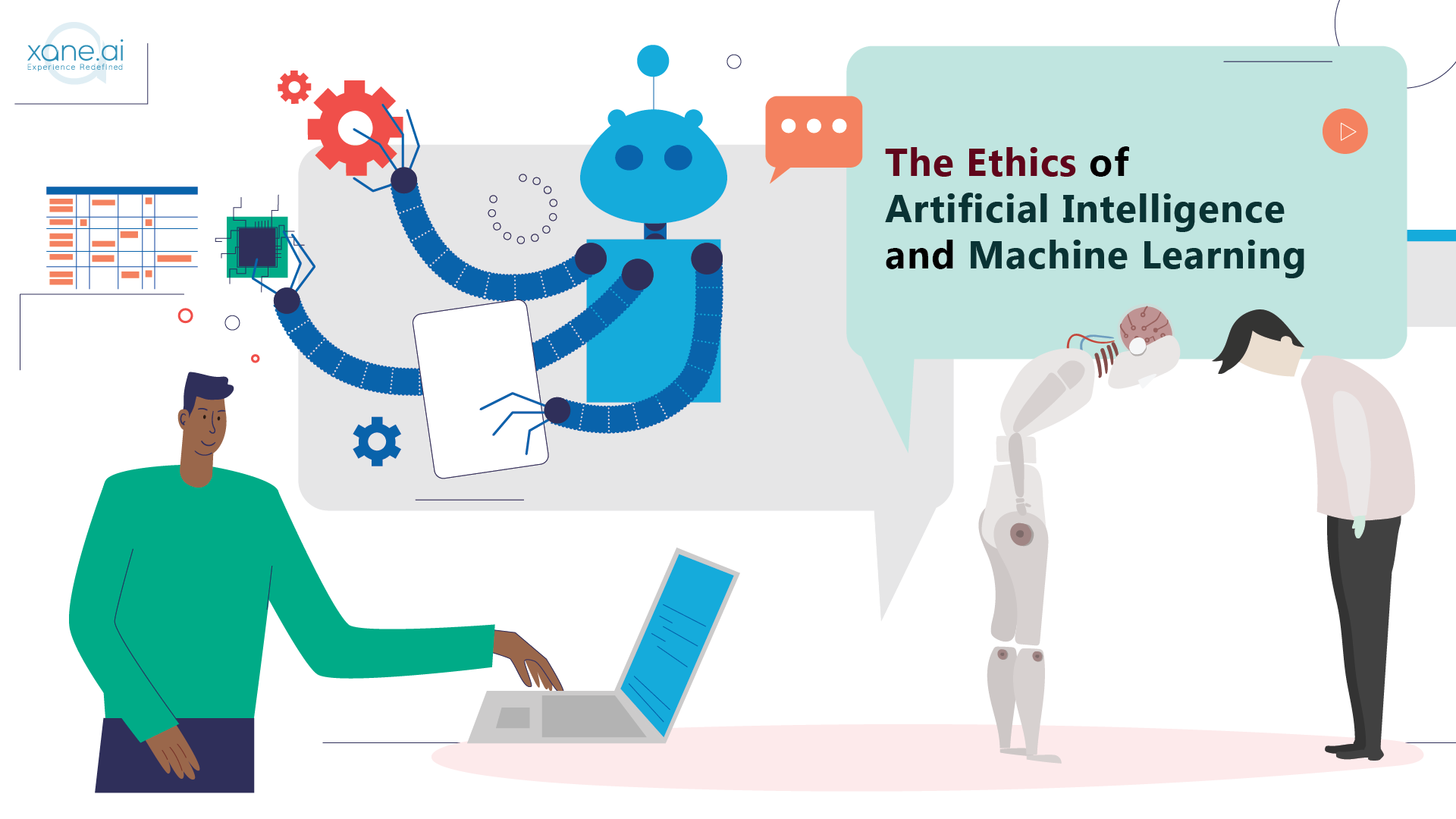
28 May The Ethics of Artificial Intelligence and Machine Learning
Blog by Oluwasegun Oke
Artificial intelligence and machine learning solutions have left their indelible mark on the digital landscape, and the stability of human existence, and will most likely continue to dictate the pace at which intelligent machines are deployed. Even with rising ethical concerns and public debates on how machine learning models mechanisms analyse and process data sets, while in automation mode – a mystery that even many experts in the computer science field have failed to unravel. The fear is that this dark side (the self-driven phase) of its operation poses a potential risk in the abundance of already commercial-scale innovations, and must be looked upon as a disservice to humanity (according to critics).
At this point, the moral and ethical shock waves cannot be ignored, so concession is that they must continually be weighed against any emerging inaccuracy and limitations, encroaching from some unreliable predictive model results (outputs), which include fundamental biases, surveillance, privacy, autonomous issues, and so on.
At this junction, this article seeks to understand why AI and ML fields are yet to reach their full potential and likewise why some designs fail to find answers to developing ethical concerns surrounding new advancements of AI-related technologies in all segments of our society
A Theory on How Machine Learning Works
We understand that AI is a technology which is aimed at giving machines all the cognitive abilities of man, such that they perform repetitive and mundane tasks with great accuracy, optimum efficiency, and advanced intelligence. Hence, we recognise machine learning as a subset of AI, likewise as a trait-simulating tool with clearly defined fields to further improve any prospect of transforming the way man and computer interact. And it is pertinent to note that the functionality milestone of this amazing technology can be fully understood from a black box point of view.
Undoubtedly, this black box contains data, which is equivalent to a set of questions and answers in broad-ranging categories and contexts, which are defined using rule-based approaches, and manipulation of models, to increase the accuracy of predictions, using simulations to find and respond to different patterns, thus providing initial commands with answers of the highest quality
Although the above feat can be achieved by creating as many black boxes as possible, to settle for those with the most reliable models, this process requires a vast amount of training data sets, including the right algorithm to attain.
Most importantly, the same algorithm continues to learn from each data and can power different versions of black boxes, which are usually mass-produced to promote optimal precision in machines’ decision-making process. As a result of this, only a black box of the highest quality (accuracy) is picked to showcase simulated machine learning capabilities, at any point in time.
The Three Challenges Inherent in the Use of Machine Learning (ML)
Data
We already know that engaging in any worthwhile machine learning innovation requires a humongous volume of data. And yes, we say data is everywhere, but do you know that only a limited number of engineers with information and access to open-sourced and free data repositories can take full advantage of data’s associated resourcefulness? A fate that also applies only to parties with the means to buy from publicly advertised data sets or any group of private enterprises
And what if at any point in tune, the available open-sourced free data, is not included to be added to your options, and your project is seeking to transform millions of lives leveraging top-of-the-chain uniquely designed algorithm models?
In this case, commercial scrambles for any missing data could portend the most horrifying and catastrophic embarkments, which end up casting an irreversibly blind spot on the need for ethics and moral justification.
The Algorithm
Thankfully in most cases, algorithms are free to access, acquire, and applied to any innovation. But what if you are facing a crisis concerning instability and the unavailability of a few models in that innovation? In essence, your present models are lacking depth (fall short in quality) and are incapable of enhancing implementation and deployment.
This amounts to another case study similar to the earlier unavailability scenario of data. In this case, your investment is seeking to lean on, to facilitate a worthwhile, successful, and sustainable business promotion.
What is then playing out may quickly turn unscrupulous engineers towards going to extreme lengths to acquire the missing models, to save the face of their investment.
Copyright infringement heavily cut across many tech giants’ networks, since projects already invested in must be completed, and this presents us with many clues to building our understanding of why increasing theft of predictive models might in most cases seem humane.
Moreso, in a unique case where a missing model seems uninspiring to acquire, and to add that if any system is deployed without its missing models, the operations usually prove abortive, and costly, by, for instance, deviating from certain functions.
This is the same reason a facility maintenance algorithm fails to alert operating personnel at the site about developing faults in critical aspects of such an infrastructure, hence posing a great risk to the workforce, who are ignorant of this threat.
The Results
The result correlates to how the accuracy of models is reported, within any network. And of the ways, individuals cheat is through training and testing of their models on datasets. It is called overfitting the dataset and amounts to a double standard, Eve as it constitutes an unwholesome practice to the parties involved.
Because in so doing (training and testing a model on the same data), the model has got an ample scale of information about the essential parameters of such dataset, making the report of its accuracy a disservice to the ethical aspect of building an ML system of the highest quality.
In the same vein, some individuals get to cheat creating synthetic data on which their respective models are trained and tested to report various anticipated levels of accuracy. Ultimately, their efforts are sabotaged by the discrepancies in leaderboards, which show much higher scores but uncorrelated results. It in essence constitutes a poor practice pushing a view to suggest unethical answers for potential computational advancements.